< Back to Sanius content
Translating Real-World Wearables and Digital Self-Reporting Data to Novel Research Insights | Vaso-Occlusive Crisis Predictions and Treatment Pathway Insights in Sickle Cell Disease
The onset of a vaso-occlusive crisis (VOC) is an all too familiar feeling for many patients with sickle cell disease (SCD) – these severe pain crises presenting as one of the most common clinical manifestations of the disease.
With each crisis, the risk of end-organ damage and a shortened lifespan grows for patients in the long-term. Indeed, an estimated 95% of SCD hospitalisations are believed to be due to VOCs, and a recent study published in Paediatric Blood and Cancer found that children hospitalised due to a VOC can continue to experience adverse effects up to 12 months after the event. Critically, a significant decrease in Health-Related Quality of Life (HRQoL) was reported most prominently after 3 months – physical functioning presenting as the most affected subscale.
With the recent revoking of conditional approval for one SCD therapy, and ongoing efforts to meet the outstanding points of query from the National Institute for Health and Care Excellence (NICE) for another, it remains as vital as ever to find alternative routes to supporting patients with the management of these crises. In light of this, our recent work has focused on research that accelerates understanding across two key fronts: the prediction of VOCs, and the characterisation of pathways for current standards of care to support the optimal positioning of novel interventions.
Due to be presented in both poster and oral presentation format later this month at the Annual Scientific Conference on Sickle Cell and Thalassaemia (ASCAT), we wanted to share an initial snapshot of this work with you here.
Predictive Technology, and the Core Variables That Feed It.
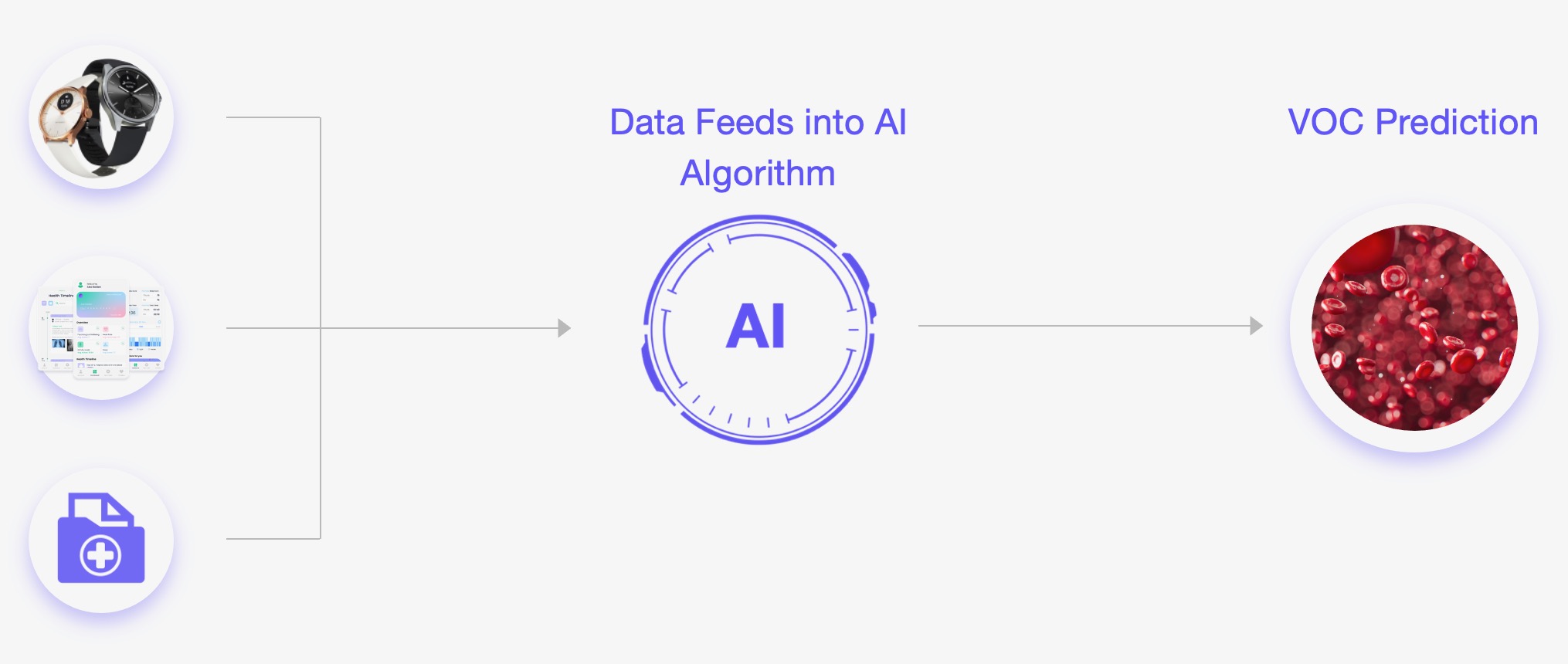
The foundation of our patient-driven ecosystem is powerful, real-world, real-time data that encompasses not only the physiological metrics found in medical records and captured by clinically validated wearable devices, but also the first-hand experiences of patients. This depth of data has allowed the development of a novel Artificial Intelligence (AI)-driven algorithm designed to predict the potential onset of a VOC, utilising longitudinal patient-reported outcomes (PRO), wearable smartwatch, and medical record data.
While each data point feeds into the predictive algorithm, with the exception of self-confirming or ‘a posteriori’ variables, a number of key variables of importance were identified. These metrics represented those with the highest importance score within the model based upon how often the variable was used in the neural net and gradient boosting machine. Focusing on the top 10, these ranged most prominently across quality of life-linked PROs, patient characteristics such as age, and environmental factors.
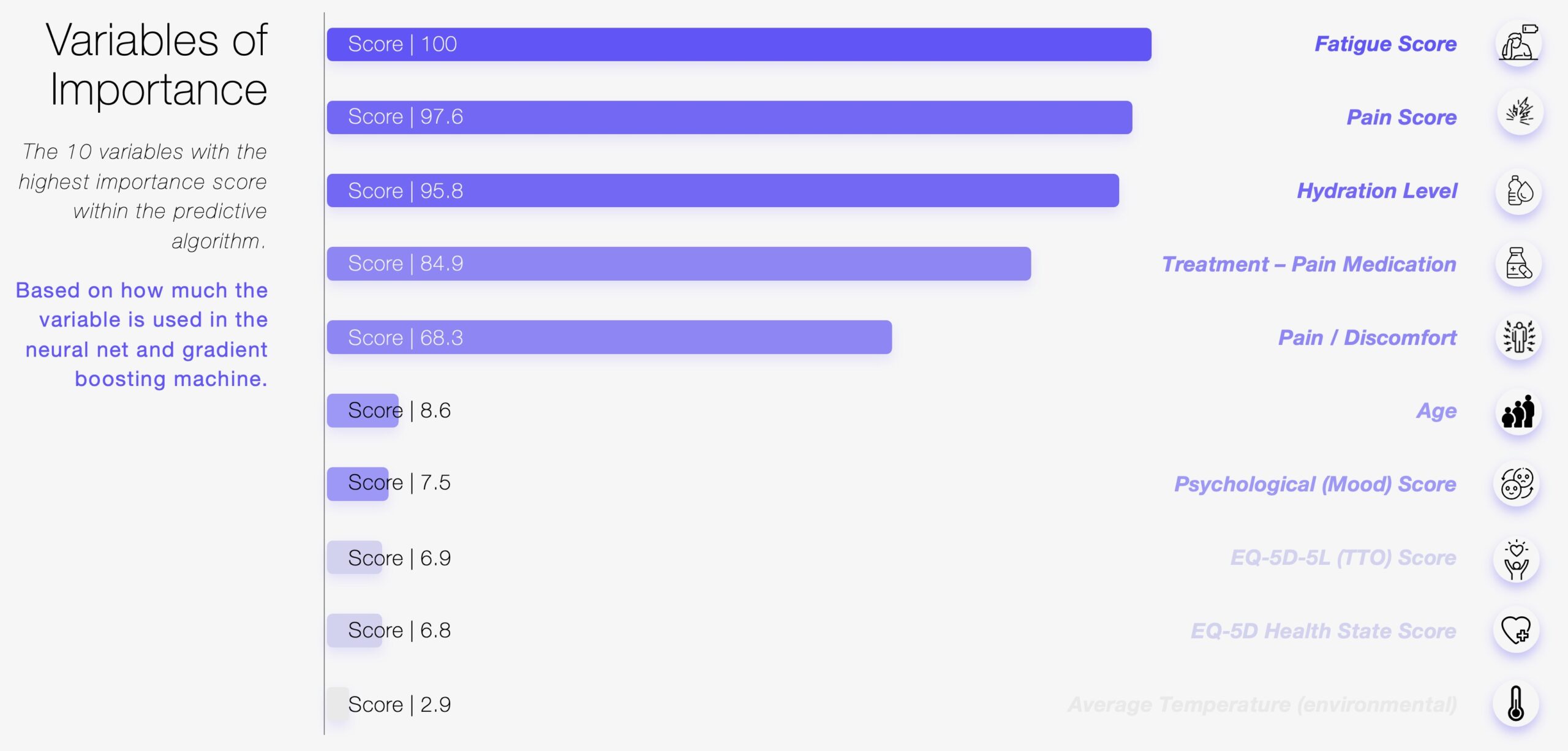
This recent piece focused on a data extract captured over 3.5 months, encompassing almost 190k datapoints across 90 distinct variables. From this, each patient’s likelihood of a VOC was calculated by the model, creating a risk score scaled from 0-100% each day. With a percentage of 75% and above considered a predicted VOC, the predictive output was assessed against the true incidence of patients’ self-reported VOCs, which were input via the mobile app each day as either a ‘Yes’, ‘Maybe’, or ‘No’.
Over the extract period, 82% of patient-reported VOCs were successfully predicted by the algorithm. Furthermore, regarding the negative predicted value, 85% of the instances in which patients reported ‘No’ VOC were predicted. As such, these insights provide an important initial translation of the daily, trackable data captured through the ecosystem to the development of real-world, VOC-predicting, AI-driven technology.
As we continue to advance this early warning system for patients and care teams to initiate proactive behavioural changes or pre-emptive interventions, our ongoing aim is to optimise the algorithm and its contributing metrics against medical data, in addition to patient self-reported events. Perhaps most importantly, this will see us explore the curation of metrics that hold the strongest predictive weighting, but require as little manual patient input as possible to reduce the burden on patients, while simultaneously improving the accuracy of the algorithm. Ultimately, we hope to provide an early alert system that will help patients and clinical teams to identify VOCs sooner, and in turn action the next steps that may prevent a possible hospital admission.
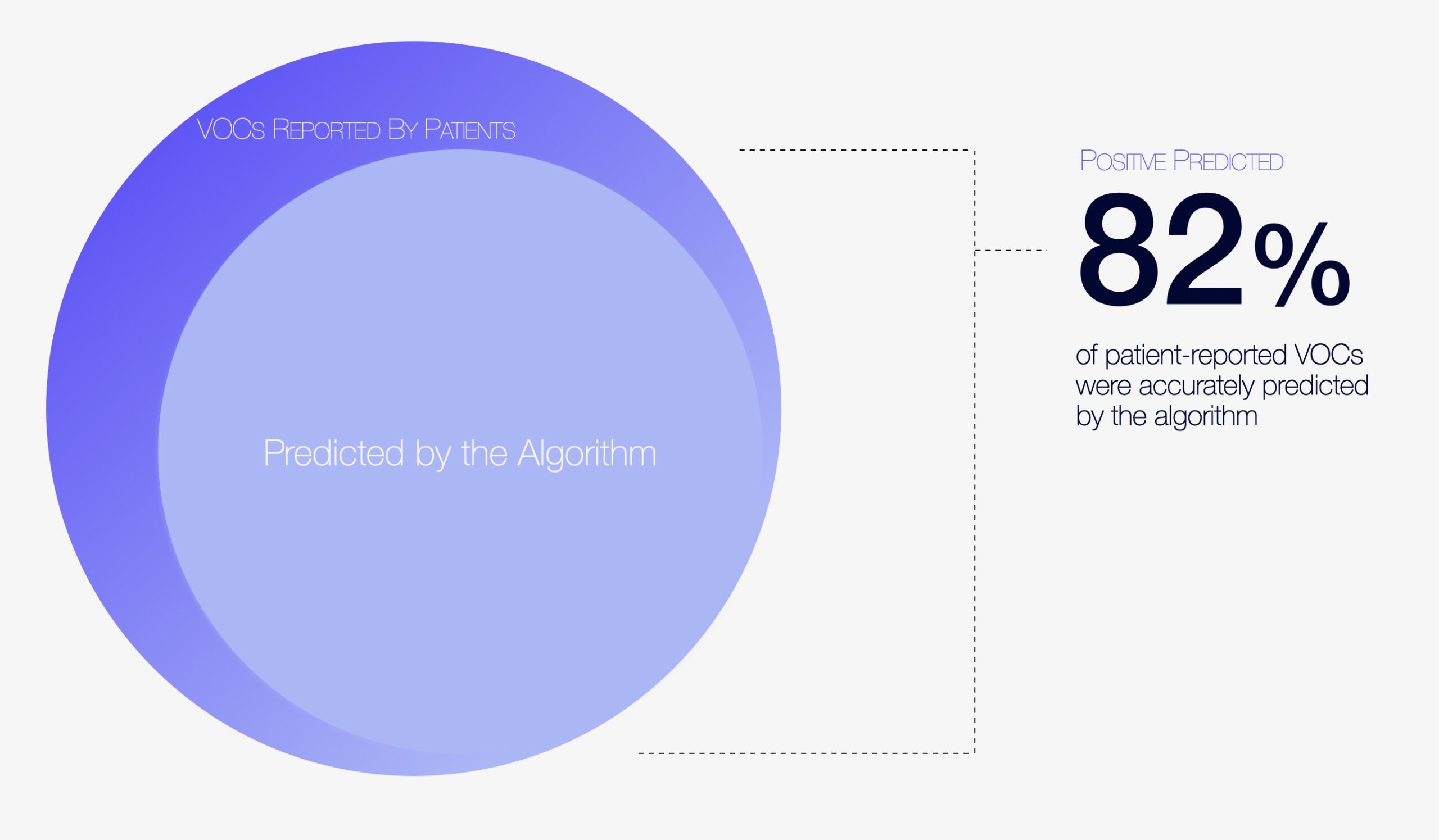
Understanding Treatment Patterns for Current Standards of Care.
A core component in generating the evidence needed to demonstrate the efficacy of novel therapies, as well as ensuring the optimal positioning in light of existing treatments, is a deep understanding of the current pathways and standards of care. This information is unfortunately frequently missing or can be poorly described, creating a significant roadblock to regulatory approvals.
As such, our second piece of work focused on better characterising this for the core interventions in SCD: transfusion therapy and hydroxyurea (HU). In particular, this sought to describe the use of transfusion therapy as a second-line treatment to HU through the comparison of data captured via Sanius’s ecosystem with aggregated patient counts and proportions, provided as snapshots by clinical teams at 4 NHS sites.
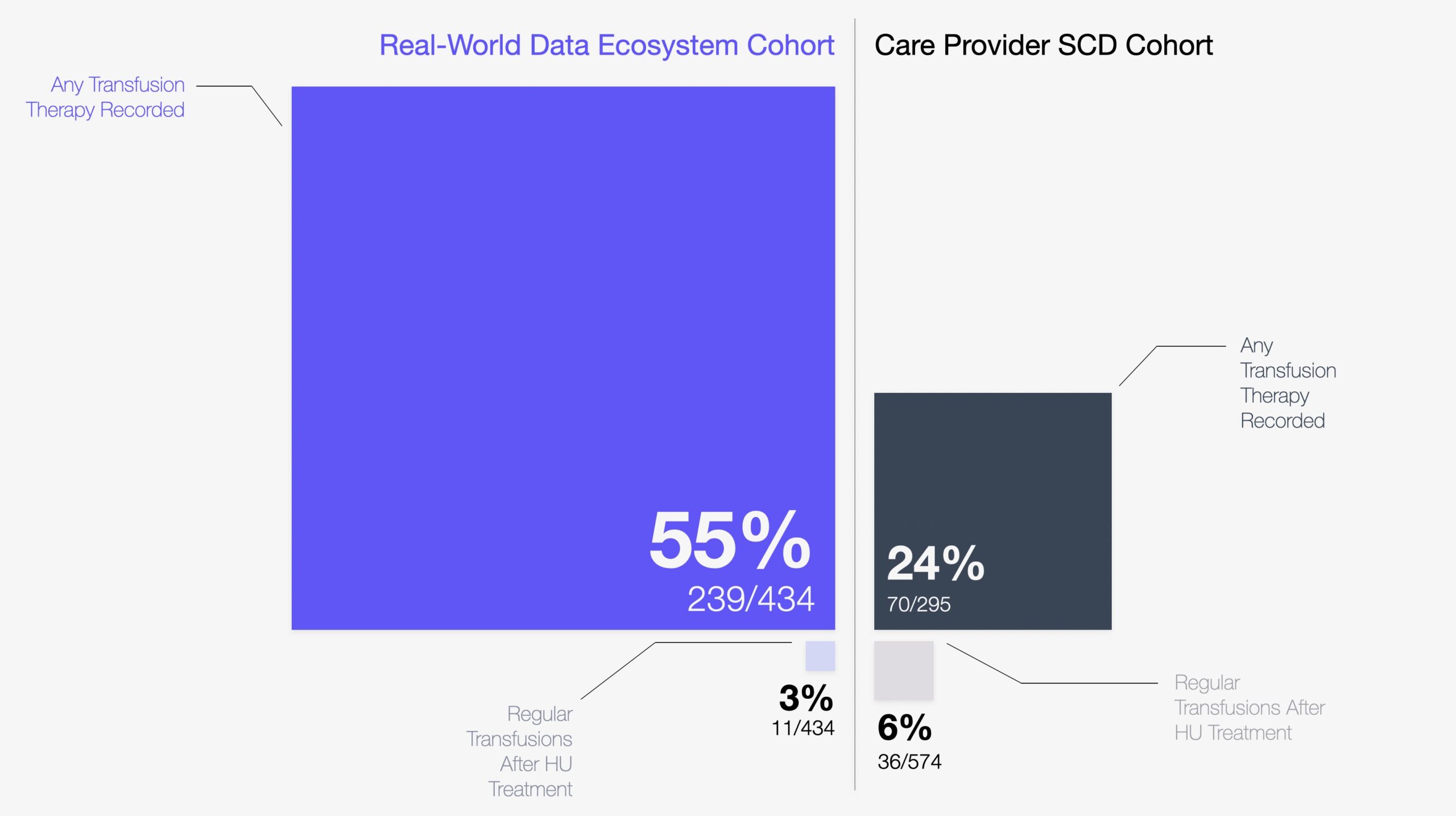
Similar proportions of patients at each treatment level were seen between the ecosystem extract and clinically reported data in regard to HU usage and the rates of HU intolerance/ineligibility. While the overall transfusion rates were notably higher in the Sanius ecosystem cohort than the care provider cohort, at 55% vs. 24%, potentially owing to the fact that not all NHS sites reported this metric, the proportion of patients receiving regular transfusion therapy after HU treatment was more closely aligned – at 3% and 6%, respectively.
With the highlighting of a significant proportion of patients who had not received HU despite being the core treatment for SCD, future work will therefore focus on exploring these pathways with deeper granularity. This will seek to potentially support an understanding of the optimal positioning of novel interventions in the real world as they emerge by developing a more detailed understanding of the treatment landscape, the proportion of patients who have not received any treatment, the underlying causes, and the resulting impact on healthcare admissions and costs.
Future Work to Accelerate Improvements to Patient Health in SCD.
Overall, our work to date has generated important real-world evidence around treatment lines across current standards of care in SCD, as a disease that continues to see very few approved treatment options. Supported by work to develop new technological advancements that support long-term health management and preventative action through predictive insights, our core goal remains the improvement of patient access to novel therapeutic options and broader approaches to monitoring their wellbeing – improving the quality of life for patients with longstanding unmet needs.
We welcome anyone who wishes to know more about our ecosystem and our work to date in real-world research insights to contact us at [email protected].
The patient experience shared.
Join the Sanius Health Community
Register your interest below and a member of our team will follow up with you soon.