< Back to Sanius content
Advancements in Real-World Healthcare-Focused AI | A Patient Use Case in Vaso-Occlusive Crisis Prediction
As we accelerate through our AI journey in improving healthcare for patients with sickle cell disease (SCD), a core focus has been tackling one of the most common clinical manifestations of the disease – vaso-occlusive crises (VOCs).
With the prospect of a potential pain crisis a near-constant concern for many patients, and with an ongoing need to keep in mind the factors that could trigger a VOC, this work forms a crucial part in advancing long-term physical and mental health for patients with SCD.
Combining automatically and manually collected wearables data – activity, sleep quality, heart rate, oxygen saturation, and ECGs – with medical record data and patient-reported outcomes (PROs) linked to quality of life (QoL), our work to date has highlighted some key variations in these metrics during a VOC. Form here, the critical next question is: how do we translate these data insights to the prediction of VOCs?
How Has the VOC Predictor Worked in Action to Date?
While commonalities certainly do exist, understanding the patient journey and deeper components that factor into the onset of a VOC requires a degree of nuance and strong visibility of each patient’s unique baselines. As such, and as we continue to progress our wider patient-driven VOC predictor pilot programme, exploration of data extracted at an individual patient level has been conducted, highlighting some interesting trends and promising output from our AI algorithm.
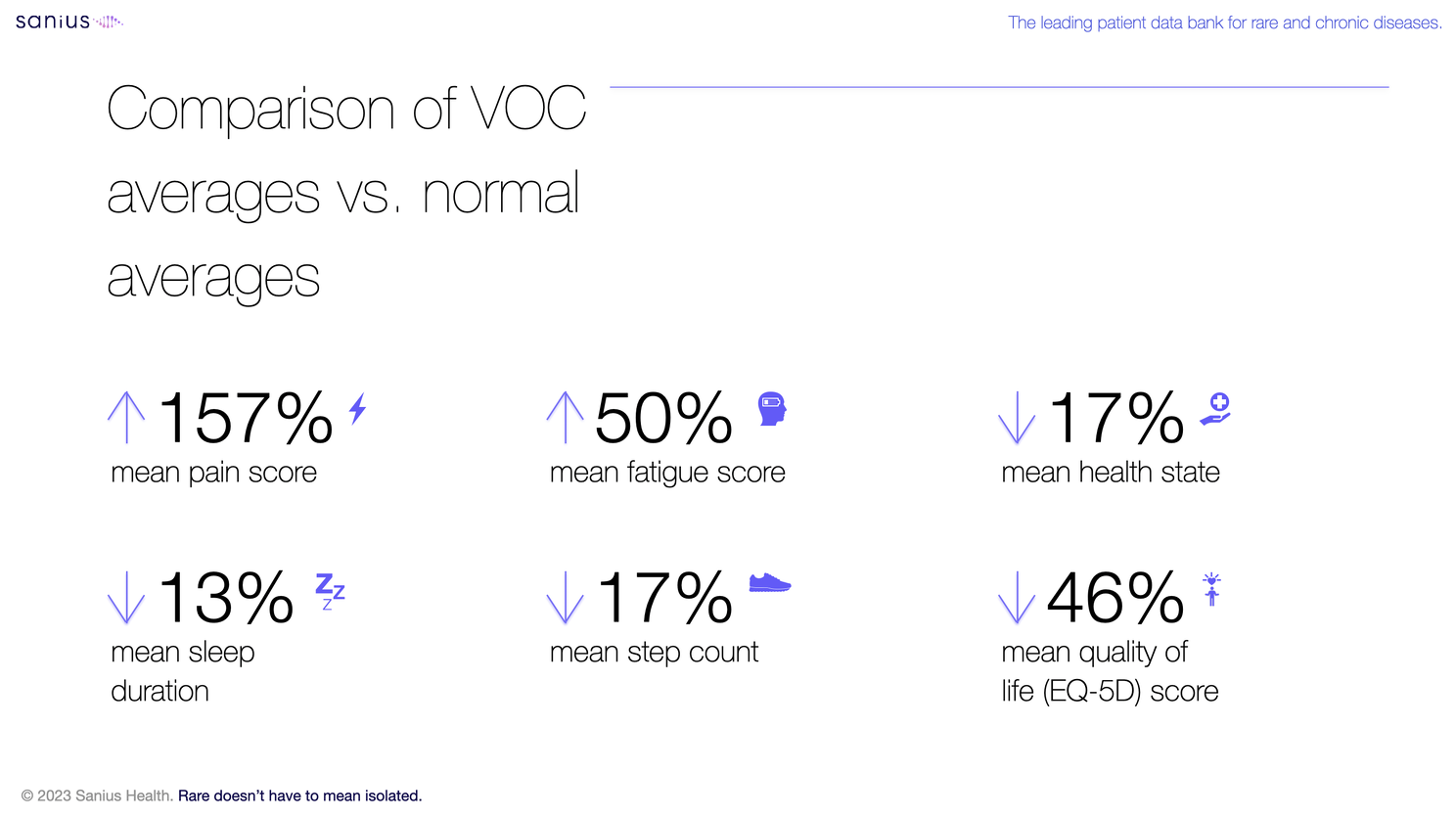
Comparing the mean scores for a selection of metrics with some of the largest variations from baseline during a VOC, a number of key variables that feed into the AI-driven VOC predictor model have been highlighted. While PROs such as perhaps more expected pain scores have been shown to soar dramatically on days where a VOC was reported, additional PROs such as fatigue scores and validated measures of QoL, the EQ-5D-5L, were also found to change significantly on these days. Moreover, automated metrics such as sleep duration and active steps were found to drop in parallel with these drops in QoL and surges in pain and fatigue.
When exploring these variables at an individual VOC event level, these increases in pain and fatigue were again seen in comparison to the patient’s typical means. This was in addition to changes in core sleep quality metrics such as deep sleep proportions and the number of nightly disturbances, or “wake up counts”. Of note, while the average activity levels calculated over the wider programme period were found to drop during a VOC, an extract of VOCs reported over the summer months appeared to show activity levels and corresponding heart rates above the patient’s usual means.
Whether this reflects an interplay between the impact of patient activity levels on the risk of a VOC and seasonal considerations is a key area of ongoing research to drive further refinement of the algorithm. Indeed, while average outdoor temperatures currently comprise one of the top 10 most important variables within the algorithm’s model, a key area of interest as we further develop the ecosystem is the capture of more local environmental factors and patients’ own skin temperatures through their wearable device.
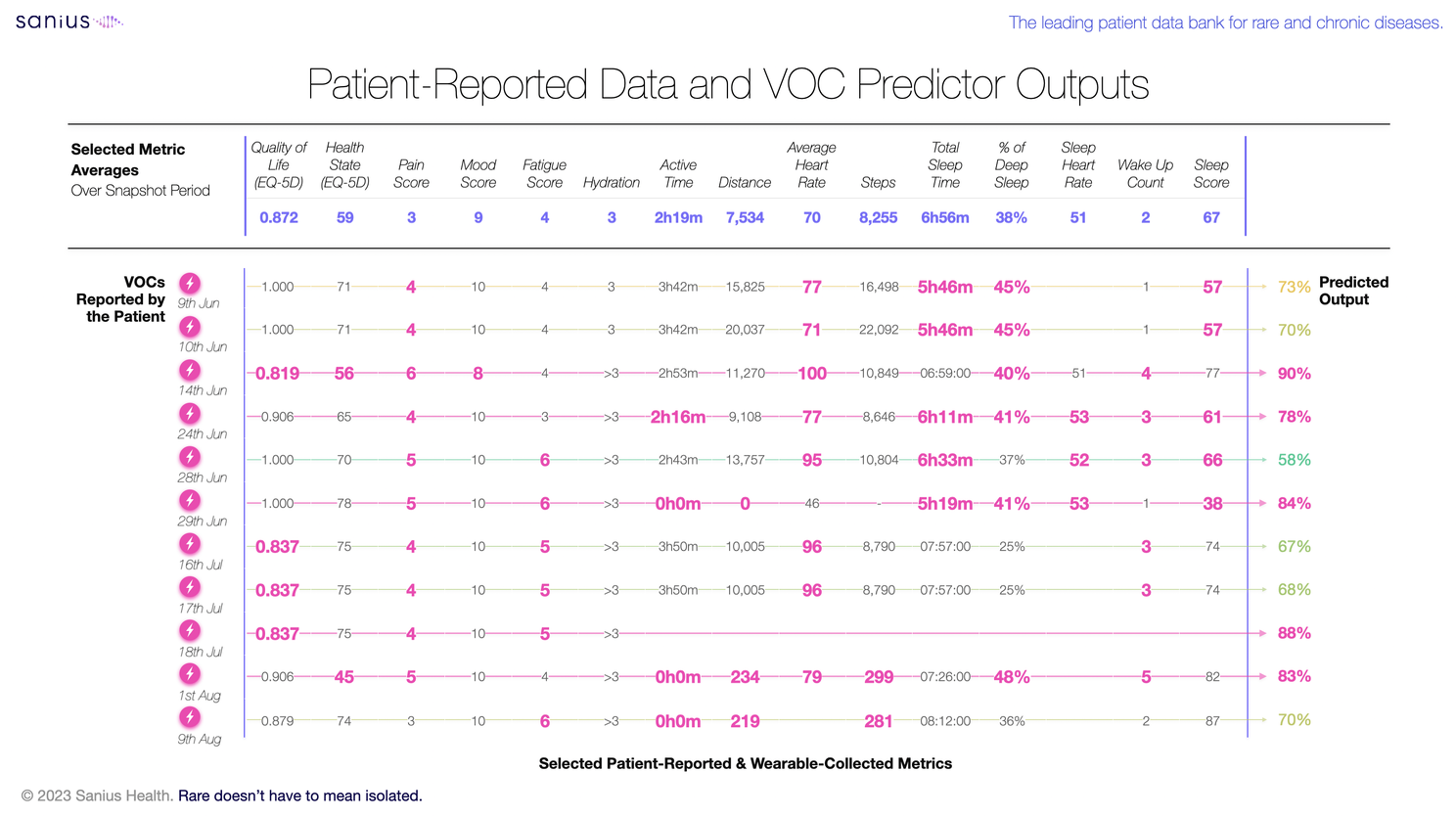
At a real-world level, snapshots from the live output of the VOC predictor pilot for the patient have seen promising correlations between highlighted metrics, predicted risk scores, and patient reports of their VOC status. Presenting actual output from the pilot predictor, drops below their typical metric averages were seen across key sleep variables when a VOC was confirmed by the patient, in line with a predicted VOC risk score above the 75% threshold. This was mirrored by a drop in activity levels and surges in pain and fatigue. Similar trends, although to a lesser degree, were seen during a potential (“maybe”) VOC reported by the patient, with an associated predicted risk score almost reaching the 75% threshold.
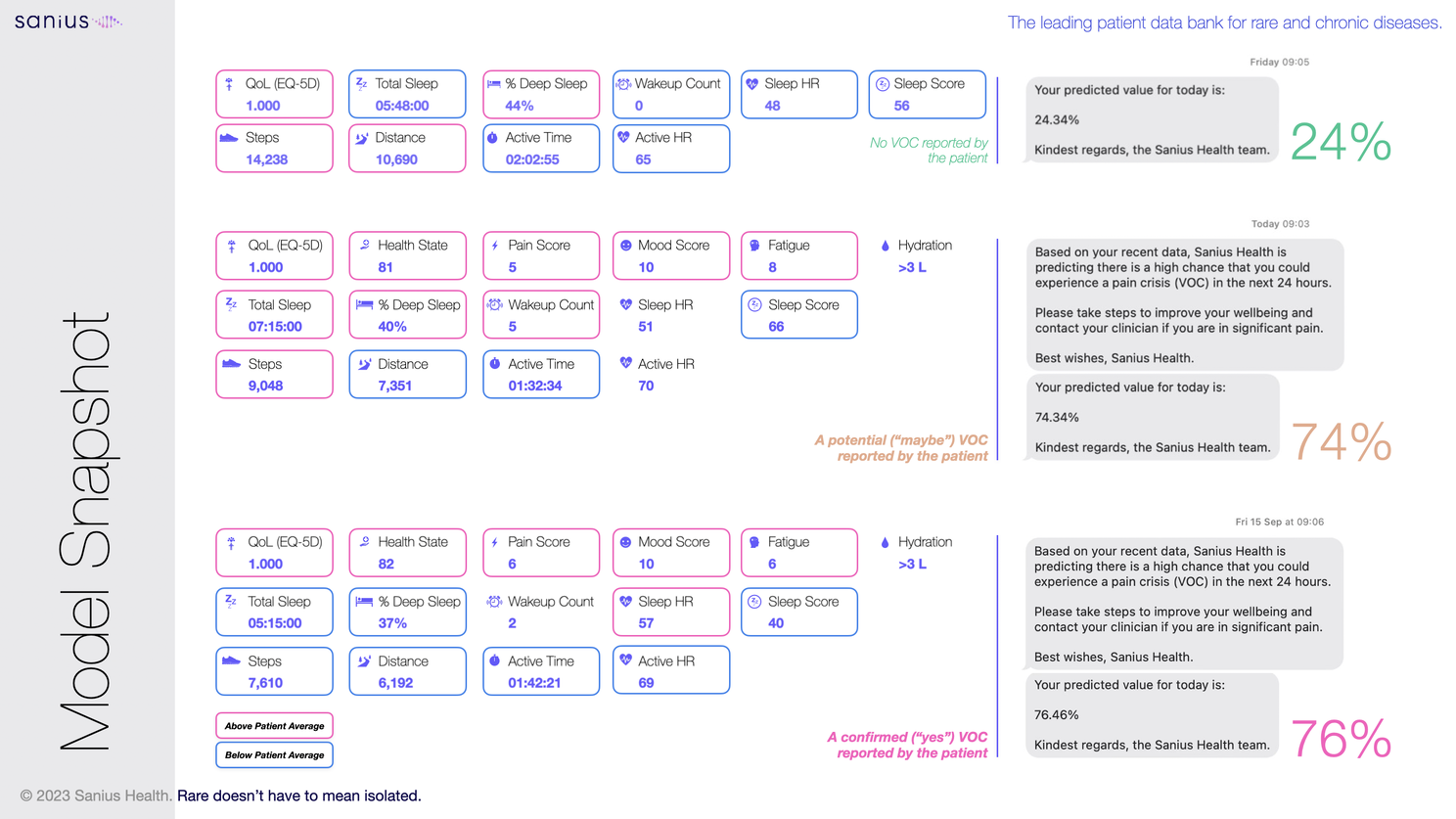
At a real-world level, snapshots from the live output of the VOC predictor pilot for the patient have seen promising correlations between highlighted metrics, predicted risk scores, and patient reports of their VOC status. Presenting actual output from the pilot predictor, drops below their typical metric averages were seen across key sleep variables when a VOC was confirmed by the patient, in line with a predicted VOC risk score above the 75% threshold. This was mirrored by a drop in activity levels and surges in pain and fatigue. Similar trends, although to a lesser degree, were seen during a potential (“maybe”) VOC reported by the patient, with an associated predicted risk score almost reaching the 75% threshold.
The patient experience shared.
Join the Sanius Health Community
Register your interest below and a member of our team will follow up with you soon.